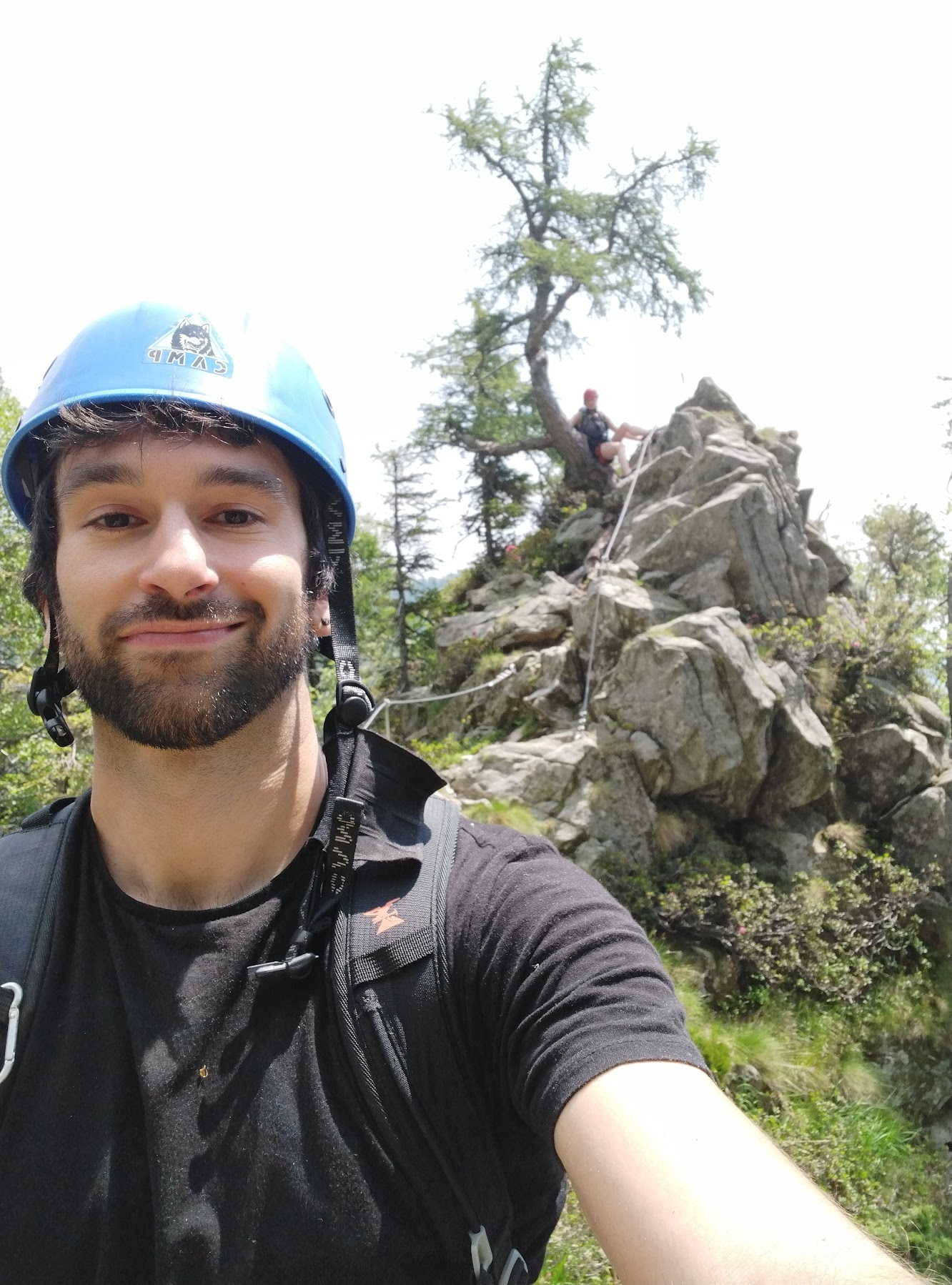
High sample complexity hampers the successful application of reinforcement learning methods, especially in real-world problems where simulating complex dynamics is computationally demanding. Influence-based abstraction (IBA) was proposed to mitigate this issue by breaking down the global model of large-scale distributed systems, such as traffic control problems, into small local sub-models. Each local model includes only a few state variables and a representation of the influence exerted by the external portion of the system. This approach allows converting a complex simulator into local lightweight simulators, enabling more effective applications of planning and reinforcement learning methods. However, the effectiveness of IBA critically depends on the ability to accurately approximate the influence of each local model. While there are a few examples showing promising results in benchmark problems, the question of whether this approach is feasible in more practical scenarios remains open. In this work, we take steps towards addressing this question by conducting an extensive empirical study of learning models for influence approximations in various realistic domains, and evaluating how these models generalize over long horizons. We find that learning the influence is often a manageable learning task, even for complex and large systems. Additionally, we demonstrate the efficacy of the approximation models for long-horizon problems. By using short trajectories, we can learn accurate influence approximations for much longer horizons.
@article{Congeduti25TMLR, title = {Influence Learning in Complex Systems}, author = {Elena Congeduti and Roberto Rocchetta and Frans A. Oliehoek}, journal = {Transactions on Machine Learning Research}, year = {2025}, url = {https://openreview.net/forum?id=tUnyInYbjK} }
This study presents a reinforcement learning framework to optimize mobility incentives in free-floating electric vehicle carsharing systems. It introduces a user-centric AI solution that assigns dynamic tariff discounts and mobility credits, learned through interaction with a simulation environment integrating demand models and historical data. The framework demonstrated improved revenues and reduced operational costs in simulations using real-world data from Tel Aviv.
@article{Rocchetta2025, title = {Optimization of Mobility Incentives in Electric Vehicle Car Sharing Systems: A Reinforcement Learning Framework}, journal = {Sustainable Cities and Society}, volume = {120}, pages = {106107}, year = {2025}, doi = {10.1016/j.scs.2024.106107}, author = {Roberto Rocchetta and Lorenzo Nespoli and Vasco Medici and Aviva Shemesh and Yael Parag and Jalomi Maayan Tardif} }
Hybrid Prognostics and Health Management (PHM) frameworks for light-emitting diodes (LEDs) seek accurate remaining useful life (RUL) predictions by merging information from physics-of-failure laws with data-driven models and tools for online monitoring and data collection. Uncertainty quantification (UQ) and uncertainty reduction are essential to achieve accurate predictions and assess the effect of heterogeneous operational-environmental conditions, lack of data, and noises on LED durability. Aleatory uncertainty is considered in hybrid frameworks, and probabilistic models and predictions are applied to account for inherent variability and randomness in the LED lifetime. On the other hand, hybrid frameworks often neglect epistemic uncertainty, lacking formal characterization and reduction methods. In this survey, we propose an overview of accelerated data collection methods and modeling options for LEDs …
@article{ROCCHETTA2024115399, title = {A survey on LED Prognostics and Health Management and uncertainty reduction}, journal = {Microelectronics Reliability}, volume = {157}, pages = {115399}, year = {2024}, issn = {0026-2714}, doi = {https://doi.org/10.1016/j.microrel.2024.115399}, author = {Roberto Rocchetta and Elisa Perrone and Alexander Herzog and Pierre Dersin and Alessandro {Di Bucchianico}}, keywords = {Light emitting diodes, Epistemic uncertainty, Prognostics and Health Management, Uncertainty quantification, Accelerated tests, Optimal design of experiment}, }
Lifetime analyses are crucial for ensuring the durability of new Light-emitting Diodes (LEDs) and uncertainty quantification (UQ) is necessary to quantify a lack of usable failure and degradation data. This work presents a new framework for predicting the lifetime of LEDs in terms of lumen maintenance, effectively quantifying the natural variability of lifetimes (aleatory) as well as the reducible uncertainty resulting from data scarcity (epistemic). Non-parametric survival models are employed for UQ of low-magnitude failures, while a new parametric interval prediction model (IPM) is introduced to characterize the uncertainty in high-magnitude lumen depreciation events and long-term extrapolated lifetimes. The width of interval-valued predictions reflects the inherent variability in degradation paths whilst the epistemic uncertainty, arising from data scarcity, is quantified by a statistical bound on the probability of the prediction errors for future degradation trajectories. A modified exponential flux decay model combined with the Arrhenius equation equips the IPM with physical information on the physics of LED luminous flux degradation. The framework is tested and validated on a novel database of LED degradation trajectories and in comparison to well-established probabilistic predictors. The results of this study support the validity of the proposed approach and the usefulness of the additional UQ capabilities.
@article{ROCCHETTA2024109715, title = {Uncertainty analysis and interval prediction of LEDs lifetimes}, journal = {Reliability Engineering & System Safety}, volume = {242}, pages = {109715}, year = {2024}, issn = {0951-8320}, doi = {https://doi.org/10.1016/j.ress.2023.109715}, author = {Roberto Rocchetta and Zhouzhao Zhan and Willem Dirk {van Driel} and Alessandro {Di Bucchianico}}, keywords = {Light-emitting Diodes, Lifetime, Lumen maintenance, Uncertainty Quantification, Accelerated Degradation Data, Interval Prediction},
A novel financial metric denominated unit financial impact indicator (UFII) is proposed to minimize the payback period for solar photovoltaic (PV) systems investments and quantify the financial efficiency of allocation and sizing strategies. However, uncontrollable environmental conditions and operational uncertainties, such as variable power demands, component failures, and weather conditions, can threaten the robustness of the investment, and their effect needs to be accounted for. Therefore, a new probabilistic framework is proposed for the robust and optimal positioning and sizing of utility-scale PV systems in a transmission network. The probabilistic framework includes a new cloud intensity simulator to model solar photovoltaic power production based on historical data and quantified using an efficient Monte Carlo method. The optimized solution obtained using weighted sums of expected UFII and its variance is compared against those obtained by using well-established economic metrics from literature. The efficiency and usefulness of the proposed approach are tested on the 14-bus IEEE power grid case study. The results prove the applicability and efficacy of the new probabilistic metric to quantify the financial effectiveness of solar photovoltaic investments on different nodes and geographical regions in a power grid, considering the unavoidable conditional and operational uncertainty.
@Article{su151511715, AUTHOR = {Cangul, Ozcel and Rocchetta, Roberto and Fahrioglu, Murat and Patelli, Edoardo}, TITLE = {Optimal Allocation and Sizing of Decentralized Solar Photovoltaic Generators Using Unit Financial Impact Indicator}, JOURNAL = {Sustainability}, VOLUME = {15}, YEAR = {2023}, NUMBER = {15}, ARTICLE-NUMBER = {11715}, URL = {https://www.mdpi.com/2071-1050/15/15/11715}, ISSN = {2071-1050}, ABSTRACT = {A novel financial metric denominated unit financial impact indicator (UFII) is proposed to minimize the payback period for solar photovoltaic (PV) systems investments and quantify the financial efficiency of allocation and sizing strategies. However, uncontrollable environmental conditions and operational uncertainties, such as variable power demands, component failures, and weather conditions, can threaten the robustness of the investment, and their effect needs to be accounted for. Therefore, a new probabilistic framework is proposed for the robust and optimal positioning and sizing of utility-scale PV systems in a transmission network. The probabilistic framework includes a new cloud intensity simulator to model solar photovoltaic power production based on historical data and quantified using an efficient Monte Carlo method. The optimized solution obtained using weighted sums of expected UFII and its variance is compared against those obtained by using well-established economic metrics from literature. The efficiency and usefulness of the proposed approach are tested on the 14-bus IEEE power grid case study. The results prove the applicability and efficacy of the new probabilistic metric to quantify the financial effectiveness of solar photovoltaic investments on different nodes and geographical regions in a power grid, considering the unavoidable conditional and operational uncertainty.}, DOI = {10.3390/su151511715} }
This work investigates formal generalization error bounds that apply to support vector machines (SVMs) in realizable and agnostic learning problems. We focus on recently observed parallels between probably approximately correct (PAC)-learning bounds, such as compression and complexity-based bounds, and novel error guarantees derived within scenario theory. Scenario theory provides nonasymptotic and distributional-free error bounds for models trained by solving data-driven decision-making problems. Relevant theorems and assumptions are reviewed and discussed. We propose a numerical comparison of the tightness and effectiveness of theoretical error bounds for support vector classifiers trained on several randomized experiments from 13 real-life problems. This analysis allows for a fair comparison of different approaches from both conceptual and experimental standpoints. Based on the numerical results, we argue that the error guarantees derived from scenario theory are often tighter for realizable problems and always yield informative results, i.e., probability bounds tighter than a vacuous [0,1] interval. This work promotes scenario theory as an alternative tool for model selection, structural-risk minimization, and generalization error analysis of SVMs. In this way, we hope to bring the communities of scenario and statistical learning theory closer, so that they can benefit from each other’s insights.
@ARTICLE{10250820, author={Rocchetta, Roberto and Mey, Alexander and Oliehoek, Frans A.}, journal={IEEE Transactions on Neural Networks and Learning Systems}, title={A Survey on Scenario Theory, Complexity, and Compression-Based Learning and Generalization}, year={2023}, volume={}, number={}, pages={1-15}, keywords={Data models;Complexity theory;Numerical models;Statistical learning;Decision making;Support vector machine classification;Picture archiving and communication systems;Agnostic learning;compression;generalization theory;probably approximately correct (PAC);scenario optimization;support vector classifiers}, doi={10.1109/TNNLS.2023.3308828}}
Fault detection models play a fundamental role in monitoring the health state of engineering systems subject to degradation processes. Data-driven fault detection models, albeit very effective when trained on large databases of failures, fail to perform well under a lack of failure examples. Because reliable engineering systems seldom fail, data shortage is often inevitable. To overcome failure data scarcity, this work proposes a new model selection framework for the robust selection of fault detection and system health monitoring models. We combine heterogeneous sources of information (time-to-failure and sensors data), a model of the system structure, and mathematical bounds on the sensitivity and specificity of components fault classifiers. We use Support Vector Machines (SVMs) to detect components faults and Scenario theory to derive formal sensitivity and specificity bounds. The component predictions are combined within a system structure-function for system health states estimation. A novel model selection strategy optimizes the hyper-parameters of the SVM ensemble by minimizing system-level prediction errors and generalization error bounds of the individual fault classifiers. One of the main advantages of the proposed framework is a set of formal epistemic bounds on fault detection and false alarm probabilities quantifying the lack of data uncertainty affecting the fault detection rate. We test the method on three representative case studies: (1) On randomized fault detection experiments with synthetic data, (2) on ten SVM models for predictive maintenance of industrial health care imaging systems, and (3) on a real-world PHM challenge problem. The results prove the efficacy of the proposed approach and its usefulness for solving fault detection.
@article{ROCCHETTA2022105140, title = {A robust model selection framework for fault detection and system health monitoring with limited failure examples: Heterogeneous data fusion and formal sensitivity bounds}, journal = {Engineering Applications of Artificial Intelligence}, volume = {114}, pages = {105140}, year = {2022}, issn = {0952-1976}, doi = {https://doi.org/10.1016/j.engappai.2022.105140}, url = {https://www.sciencedirect.com/science/article/pii/S0952197622002627}, author = {Roberto Rocchetta and Qi Gao and Dimitrios Mavroeidis and Milan Petkovic}, keywords = {PHM, Fault detection, Sensitivity bounds, Information fusion, SVM, System health monitoring}}
Controlled islanding can enhance power grid resilience and help mitigate the effect of emerging failure by splitting the grid into islands that can be rapidly and independently recovered and managed. In practice, controlled islanding is challenging and requires vulnerability assessment and uncertainty quantification. In this work, we investigate robustness drops due to line failures and a controlled partitioning strategy for mitigating their consequences. A spectral clustering algorithm is employed to decompose the adjacency matrix of the damaged network and identify optimal network partitions. The adjacency matrix summarizes the power system topology, and different dynamic and static electrical factors such as line impedance and flows are employed to weigh the importance of the grid's cables. Differently from other works, we propose a statistical correlation analysis between vulnerability metrics and goodness of cluster scores. We investigate expected trends in the scores for randomized contingencies of increasing orders and examine their variability for random outages of a given size. We observed that the spectral radius and natural connectivity vary less on randomized failure events of a given size and are more sensitive to the selection of the adjacency matrix weights. Vulnerability scores based on the algebraic connectivity have a higher coefficient of variation for a given damage size and are less dependent on the specific dynamic and static electrical weighting factors. We show a few consistent patterns in the correlations between the scores for the vulnerability of the grid and the optimal clusters. The strength and sign of the correlation coefficients depend on the different electrical factors weighting the transmission lines and the grid-specific topology.
@article{ROCCHETTA2022112185, title = {Enhancing the resilience of critical infrastructures: Statistical analysis of power grid spectral clustering and post-contingency vulnerability metrics}, journal = {Renewable and Sustainable Energy Reviews}, volume = {159}, pages = {112185}, year = {2022}, issn = {1364-0321}, doi = {https://doi.org/10.1016/j.rser.2022.112185}, url = {https://www.sciencedirect.com/science/article/pii/S1364032122001095}, author = {Roberto Rocchetta}, keywords = {Resilience, Vulnerability, Power grid, contingencies, Spectral clustering, Networks topology}}
In practical engineering, experimental data is not fully in line with the true system response due to various uncertain factors, e.g., parameter imprecision, model uncertainty, and measurement errors. In the presence of mixed sources of aleatory and epistemic uncertainty, stochastic model updating is a powerful tool for model validation and parameter calibration. This paper investigates the use of Bray-Curtis (B-C) distance in stochastic model updating and proposes a Bayesian approach addressing a scenario where the dataset contains multiple outliers. In the proposed method, a B-C distance-based uncertainty quantification metric is employed, that rewards models for which the discrepancy between observations and simulated samples is small while penalizing those which exhibit large differences. To improve the computational efficiency, an adaptive binning algorithm is developed and embedded into the Bayesian approximate computation framework. The merit of this algorithm is that the number of bins is automatically selected according to the difference between the experimental data and the simulated data. The effectiveness and efficiency of the proposed method is verified via two numerical cases and an engineering case from the NASA 2020 UQ challenge. Both static and dynamic cases with explicit and implicit propagation models are considered.
@article{ ZHAO2022108889, title = {Enriching stochastic model updating metrics: An efficient Bayesian approach using Bray-Curtis distance and an adaptive binning algorithm}, journal = {Mechanical Systems and Signal Processing}, volume = {171}, pages = {108889}, year = {2022}, issn = {0888-3270}, doi = {https://doi.org/10.1016/j.ymssp.2022.108889}, url = {https://www.sciencedirect.com/science/article/pii/S0888327022000796}, author = {Wenhua Zhao and Lechang Yang and Chao Dang and Roberto Rocchetta and Marcos Valdebenito and David Moens}, keywords = {Bayesian inversion, Stochastic model updating, Approximate Bayesian computation, Bray-Curtis distance, Adaptive binning algorithm} }
In this paper we present a framework for addressing a variety of engineering design challenges with limited empirical data and partial information. This framework includes guidance on the characterisation of a mixture of uncertainties, efficient methodologies to integrate data into design decisions, and to conduct reliability analysis, and risk/reliability based design optimisation. To demonstrate its efficacy, the framework has been applied to the NASA 2020 uncertainty quantification challenge. The results and discussion in the paper are with respect to this application.
@article{GRAY2022108210, title = {From inference to design: A comprehensive framework for uncertainty quantification in engineering with limited information}, journal = {Mechanical Systems and Signal Processing}, volume = {165}, pages = {108210}, year = {2022}, issn = {0888-3270}, doi = {https://doi.org/10.1016/j.ymssp.2021.108210}, url = {https://www.sciencedirect.com/science/article/pii/S0888327021005859}, author = {A. Gray and A. Wimbush and M. {de Angelis} and P.O. Hristov and D. Calleja and E. Miralles-Dolz and R. Rocchetta}, keywords = {Bayesian calibration, Probability bounds analysis, Uncertainty propagation, Uncertainty reduction, Epistemic uncertainty, Optimisation under uncertainty}, abstract = {In this paper we present a framework for addressing a variety of engineering design challenges with limited empirical data and partial information. This framework includes guidance on the characterisation of a mixture of uncertainties, efficient methodologies to integrate data into design decisions, and to conduct reliability analysis, and risk/reliability based design optimisation. To demonstrate its efficacy, the framework has been applied to the NASA 2020 uncertainty quantification challenge. The results and discussion in the paper are with respect to this application.} }
Reliability-based design approaches via scenario optimization are driven by data thereby eliminating the need for creating a probabilistic model of the uncertain parameters. A scenario approach not only yields a reliability-based design that is optimal for the existing data, but also a probabilistic certificate of its correctness against future data drawn from the same source. In this article, we seek designs that minimize not only the failure probability but also the risk measured by the expected severity of requirement violations. The resulting risk-based solution is equipped with a probabilistic certificate of correctness that depends on both the amount of data available and the complexity of the design architecture. This certificate is comprised of an upper and lower bound on the probability of exceeding a value-at-risk (quantile) level. A reliability interval can be easily derived by selecting a specific quantile value and it is mathematically guaranteed for any reliability constraints having a convex dependency on the decision variable, and an arbitrary dependency on the uncertain parameters. Furthermore, the proposed approach enables the analyst to mitigate the effect of outliers in the data set and to trade-off the reliability of competing requirements.
@article{ROCCHETTA2021107900, title = {A scenario optimization approach to reliability-based and risk-based design: Soft-constrained modulation of failure probability bounds}, journal = {Reliability Engineering & System Safety}, volume = {216},pages = {107900},year = {2021},issn = {0951-8320}, doi = {https://doi.org/10.1016/j.ress.2021.107900}, url = {https://www.sciencedirect.com/science/article/pii/S095183202100418X}, author = {Roberto Rocchetta and Luis G. Crespo}, keywords = {Reliability-based design optimization, Scenario theory, Reliability bounds, Conditional value-at-risk, Constraints relaxation, Lack of data uncertainty, Convex programs}, abstract = {Reliability-based design approaches via scenario optimization are driven by data thereby eliminating the need for creating a probabilistic model of the uncertain parameters. A scenario approach not only yields a reliability-based design that is optimal for the existing data, but also a probabilistic certificate of its correctness against future data drawn from the same source. In this article, we seek designs that minimize not only the failure probability but also the risk measured by the expected severity of requirement violations. The resulting risk-based solution is equipped with a probabilistic certificate of correctness that depends on both the amount of data available and the complexity of the design architecture. This certificate is comprised of an upper and lower bound on the probability of exceeding a value-at-risk (quantile) level. A reliability interval can be easily derived by selecting a specific quantile value and it is mathematically guaranteed for any reliability constraints having a convex dependency on the decision variable, and an arbitrary dependency on the uncertain parameters. Furthermore, the proposed approach enables the analyst to mitigate the effect of outliers in the data set and to trade-off the reliability of competing requirements.} }
Interval Predictor Models (IPMs) offer a non-probabilistic, interval-valued, characterization of the uncertainty affecting random data generating processes. IPMs are constructed directly from data, with no assumptions on the distributions of the uncertain factors driving the process, and are therefore exempt from the subjectivity induced by such a practice. The reliability of an IPM defines the probability of correct predictions for future samples and, in practice, its true value is always unknown due to finite samples sizes and limited understanding of the process. This paper proposes an overview of scenario optimization programs for the identification of IPMs. Traditional IPM identification methods are compared with a new scheme which softens the scenario constraints and exploits a trade-off between reliability and accuracy. The new methods allows prescribing predictors that achieve higher accuracy for a quantifiable reduction in the reliability. Scenario optimization theory is the mathematical tool used to prescribe formal epistemic bounds on the predictors reliability. A review of relevant theorems and bounds is proposed in this work. Scenario-based reliability bounds hold distribution-free, non asymptotically, and quantify the uncertainty affecting the model’s ability to correctly predict future data. The applicability of the new approach is tested on three examples: i) on the modelling of a trigonometric function affected by a noise term, ii) on the identification of a black-box system-controller dynamic response model and, iii) on the modelling of the vibration response of a car suspension arm crossed by a crack of unknown length. The points of strength and limitations of the new IPM are discussed based on the accuracy, computational cost, and width of the resulting epistemic bounds.
@article{ROCCHETTA2021107973, title = {Soft-constrained interval predictor models and epistemic reliability intervals: A new tool for uncertainty quantification with limited experimental data}, journal = {Mechanical Systems and Signal Processing}, volume = {161}, pages = {107973}, year = {2021}, issn = {0888-3270}, doi = {https://doi.org/10.1016/j.ymssp.2021.107973}, url = {https://www.sciencedirect.com/science/article/pii/S088832702100368X}, author = {Roberto Rocchetta and Qi Gao and Milan Petkovic}, keywords = {Interval predictor models, Scenario theory, Epistemic uncertainty, Reliability bounds, Convex optimization, Frequency response function}, abstract = {Interval Predictor Models (IPMs) offer a non-probabilistic, interval-valued, characterization of the uncertainty affecting random data generating processes. IPMs are constructed directly from data, with no assumptions on the distributions of the uncertain factors driving the process, and are therefore exempt from the subjectivity induced by such a practice. The reliability of an IPM defines the probability of correct predictions for future samples and, in practice, its true value is always unknown due to finite samples sizes and limited understanding of the process. This paper proposes an overview of scenario optimization programs for the identification of IPMs. Traditional IPM identification methods are compared with a new scheme which softens the scenario constraints and exploits a trade-off between reliability and accuracy. The new methods allows prescribing predictors that achieve higher accuracy for a quantifiable reduction in the reliability. Scenario optimization theory is the mathematical tool used to prescribe formal epistemic bounds on the predictors reliability. A review of relevant theorems and bounds is proposed in this work. Scenario-based reliability bounds hold distribution-free, non asymptotically, and quantify the uncertainty affecting the model’s ability to correctly predict future data. The applicability of the new approach is tested on three examples: i) on the modelling of a trigonometric function affected by a noise term, ii) on the identification of a black-box system-controller dynamic response model and, iii) on the modelling of the vibration response of a car suspension arm crossed by a crack of unknown length. The points of strength and limitations of the new IPM are discussed based on the accuracy, computational cost, and width of the resulting epistemic bounds.
}Risk-based power dispatch has been proposed as a viable alternative to Security-Constrained Dispatch to reduce power grid costs and help to better understand of prominent hazards. In contrast to classical approaches, risk-based frameworks assign different weights to different contingencies, quantifying both their likelihood occurrence and severity. This leads to an economically profitable operational schedule by exploiting the trade-off between grid risks and costs. However, relevant sources of uncertainty are often neglected due to issues related to the computational cost of the analysis. In this work, we present an efficient risk assessment frameworks for power grids. The approach is based on the Line-Outage Distribution Factors for the severity assessment of post-contingency scenarios. The proposed emulator is embedded within a generalized uncertainty quantification framework to quantify: (1) The effect of imprecision on the estimation of the risk index; (2) The effect of inherent variability, aleatory uncertainty, in environmental-operational variables. The computational cost and accuracy of the proposed risk model are discussed in comparison to traditional approaches. The applicability of the proposed framework to real size grids is exemplified by several case studies.
@article{ROCCHETTA2020106817, title = "A post-contingency power flow emulator for generalized probabilistic risks assessment of power grids", journal = "Reliability Engineering & System Safety", volume = "197", pages = "106817", year = "2020", issn = "0951-8320", doi = "https://doi.org/10.1016/j.ress.2020.106817", url = "http://www.sciencedirect.com/science/article/pii/S0951832019303023", author = "Roberto Rocchetta and Edoardo Patelli"}
This article introduces a scenario optimization framework for reliability-based design given a set of observations of uncertain parameters. In contrast to traditional methods, scenario optimization makes direct use of the available data thereby eliminating the need for creating a probabilistic model of the uncertainty in the parameters. This feature makes the resulting design exempt from the subjectivity caused by prescribing an uncertainty model from insufficient data. Furthermore, scenario theory enables rigorously bounding the probability of the resulting design satisfying the reliability requirements imposed upon it with respect to additional, unseen observations drawn from the same data-generating-mechanism. This bound, which is non-asymptotic and distribution-free, requires calculating the set of support constraints corresponding to the optimal design. In this paper we propose a framework for seeking such a design and a computationally tractable algorithm for calculating such a set. This information allows determining the degree of stringency that each individual requirement imposes on the optimal design. Furthermore, we propose a chance-constrained optimization technique to eliminate the effect of outliers in the resulting optimal design. The ideas proposed are illustrated by a set of easily reproducible case studies having algebraic limit state functions.
@article{ ROCCHETTA2020106755, title = "A scenario optimization approach to reliability-based design", journal = "Reliability Engineering & System Safety", volume = "196", pages = "106755", year = "2020", issn = "0951-8320", doi = "https://doi.org/10.1016/j.ress.2019.106755", url = "http://www.sciencedirect.com/science/article/pii/S0951832019309639", author = "Roberto Rocchetta and Luis G. Crespo and Sean P. Kenny", keywords = "Reliability-based design optimization, Support constraints, Scenario optimization, Probability of failure, Outliers, Worst-case", abstract = "This article introduces a scenario optimization framework for reliability-based design given a set of observations of uncertain parameters. In contrast to traditional methods, scenario optimization makes direct use of the available data thereby eliminating the need for creating a probabilistic model of the uncertainty in the parameters. This feature makes the resulting design exempt from the subjectivity caused by prescribing an uncertainty model from insufficient data. Furthermore, scenario theory enables rigorously bounding the probability of the resulting design satisfying the reliability requirements imposed upon it with respect to additional, unseen observations drawn from the same data-generating-mechanism. This bound, which is non-asymptotic and distribution-free, requires calculating the set of support constraints corresponding to the optimal design. In this paper we propose a framework for seeking such a design and a computationally tractable algorithm for calculating such a set. This information allows determining the degree of stringency that each individual requirement imposes on the optimal design. Furthermore, we propose a chance-constrained optimization technique to eliminate the effect of outliers in the resulting optimal design. The ideas proposed are illustrated by a set of easily reproducible case studies having algebraic limit state functions." }
We develop a Reinforcement Learning framework for the optimal management of the operation and maintenance of power grids equipped with prognostics and health management capabilities. Reinforcement learning exploits the information about the health state of the grid components. Optimal actions are identified maximizing the expected profit, considering the aleatory uncertainties in the environment. To extend the applicability of the proposed approach to realistic problems with large and continuous state spaces, we use Artificial Neural Networks (ANN) tools to replace the tabular representation of the state-action value function. The non-tabular Reinforcement Learning algorithm adopting an ANN ensemble is designed and tested on the scaled-down power grid case study, which includes renewable energy sources, controllable generators, maintenance delays and prognostics and health management devices. The method strengths and weaknesses are identified by comparison to the reference Bellman’s optimally. Results show good approximation capability of Q-learning with ANN, and that the proposed framework outperforms expert-based solutions to grid operation and maintenance management.
@article{ROCCHETTA2019291, title = "A reinforcement learning framework for optimal operation and maintenance of power grids", journal = "Applied Energy", volume = "241", pages = "291 - 301", year = "2019", issn = "0306-2619", doi = "https://doi.org/10.1016/j.apenergy.2019.03.027", author = "R. Rocchetta and L. Bellani and M. Compare and E. Zio and E. Patelli", }
A generalised uncertainty quantification framework for resilience assessment of weather-coupled, repairable power grids is presented. The framework can be used to efficiently quantify both epistemic and aleatory uncertainty affecting grid-related and weather-related factors. The power grid simulator has been specifically designed to model interactions between severe weather conditions and grid dynamic states and behaviours, such as weather-induced failures or delays in components replacements. A resilience index is computed by adopting a novel algorithm which exploits a vectorised emulator of the power-flow solver to reduce the computational efforts. The resilience stochastic modelling framework is embedded into a non-intrusive generalised stochastic framework, which enables the analyst to quantify the effect of parameters imprecision. A modified version of the IEEE 24 nodes reliability test system has been used as representative case study. The surrogate-based model and the Power-Flow-based model are compared, and the results show similar accuracy but enhanced efficiency of the former. Global sensitivity of the resilience index to increasing imprecision in parameters of the probabilistic model has been analysed. The relevance of specific weather/grid uncertain factors is highlighted by global sensitivity analysis and the importance of dealing with imprecision in the information clearly emerges.
@article{ROCCHETTA2018339, title = "A power-flow emulator approach for resilience assessment of repairable power grids subject to weather-induced failures and data deficiency", journal = "Applied Energy", volume = "210", pages = "339 - 350", year = "2018", issn = "0306-2619", doi = "https://doi.org/10.1016/j.apenergy.2017.10.126", author = "Roberto Rocchetta and Enrico Zio and Edoardo Patelli" }
Vulnerability and robustness are major concerns for future power grids. Malicious attacks and extreme weather conditions have the potential to trigger multiple components outages, cascading failures and large blackouts. Robust contingency identification procedures are necessary to improve power grids resilience and identify critical scenarios. This paper proposes a framework for advanced uncertainty quantification and vulnerability assessment of power grids. The framework allows critical failure scenarios to be identified and overcomes the limitations of current approaches by explicitly considering aleatory and epistemic sources of uncertainty modelled using probability boxes. The different effects of stochastic fluctuation of the power demand, imprecision in power grid parameters and uncertainty in the selection of the vulnerability model have been quantified. Spectral graph metrics for vulnerability are computed using different weights and are compared to power-flow-based cascading indices in ranking N-1 line failures and random N-k lines attacks. A rank correlation test is proposed for further comparison of the vulnerability metrics. The IEEE 24 nodes reliability test power network is selected as a representative case study and a detailed discussion of the results and findings is presented.
@article{ROCCHETTA2018219, title = "Assessment of power grid vulnerabilities accounting for stochastic loads and model imprecision", journal = "International Journal of Electrical Power & Energy Systems", volume = "98", pages = "219 - 232", year = "2018", issn = "0142-0615", doi = "https://doi.org/10.1016/j.ijepes.2017.11.047", author = "Roberto Rocchetta and Edoardo Patelli" }
Fatigue induced cracks is a dangerous failure mechanism which affects mechanical components subject to alternating load cycles. System health monitoring should be adopted to identify cracks which can jeopardise the structure. Real-time damage detection may fail in the identification of the cracks due to different sources of uncertainty which have been poorly assessed or even fully neglected. In this paper, a novel efficient and robust procedure is used for the detection of cracks locations and lengths in mechanical components. A Bayesian model updating framework is employed, which allows accounting for relevant sources of uncertainty. The idea underpinning the approach is to identify the most probable crack consistent with the experimental measurements. To tackle the computational cost of the Bayesian approach an emulator is adopted for replacing the computationally costly Finite Element model. To improve the overall robustness of the procedure, different numerical likelihoods, measurement noises and imprecision in the value of model parameters are analysed and their effects quantified. The accuracy of the stochastic updating and the efficiency of the numerical procedure are discussed. An experimental aluminium frame and on a numerical model of a typical car suspension arm are used to demonstrate the applicability of the approach.
@article{ROCCHETTA2018174, title = "On-line Bayesian model updating for structural health monitoring", journal = "Mechanical Systems and Signal Processing", volume = "103", pages = "174 - 195", year = "2018", issn = "0888-3270", doi = "https://doi.org/10.1016/j.ymssp.2017.10.015", author = "Roberto Rocchetta and Matteo Broggi and Quentin Huchet and Edoardo Patelli" }
A generalised probabilistic framework is proposed for reliability assessment and uncertainty quantification under a lack of data. The developed computational tool allows the effect of epistemic uncertainty to be quantified and has been applied to assess the reliability of an electronic circuit and a power transmission network. The strength and weakness of the proposed approach are illustrated by comparison to traditional probabilistic approaches. In the presence of both aleatory and epistemic uncertainty, classic probabilistic approaches may lead to misleading conclusions and a false sense of confidence which may not fully represent the quality of the available information. In contrast, generalised probabilistic approaches are versatile and powerful when linked to a computational tool that permits their applicability to realistic engineering problems.
@article{ROCCHETTA2018710, title = "Do we have enough data? Robust reliability via uncertainty quantification", journal = "Applied Mathematical Modelling", volume = "54", pages = "710 - 721", year = "2018", issn = "0307-904X", doi = "https://doi.org/10.1016/j.apm.2017.10.020", author = "Roberto Rocchetta and Matteo Broggi and Edoardo Patelli" }
Security and reliability are major concerns for future power systems with distributed generation. A comprehensive evaluation of the risk associated with these systems must consider contingencies under normal environmental conditions and also extreme ones. Environmental conditions can strongly influence the operation and performance of distributed generation systems, not only due to the growing shares of renewable-energy generators installed but also for the environment-related contingencies that can damage or deeply degrade the components of the power grid. In this context, the main novelty of this paper is the development of probabilistic risk assessment and risk-cost optimization framework for distributed power generation systems, that take the effects of extreme weather conditions into account. A Monte Carlo non-sequential algorithm is used for generating both normal and severe weather. The probabilistic risk assessment is embedded within a risk-based, bi-objective optimization to find the optimal size of generators distributed on the power grid that minimize both risks and cost associated with severe weather. An application is shown on a case study adapted from the IEEE 13 nodes test system. By comparing the results considering normal environmental conditions and the results considering the effects of extreme weather, the relevance of the latter clearly emerges.
@article{ROCCHETTA201547, title = "Risk assessment and risk-cost optimization of distributed power generation systems considering extreme weather conditions", journal = "Reliability Engineering & System Safety", volume = "136", pages = "47 - 61", year = "2015", issn = "0951-8320", doi = "https://doi.org/10.1016/j.ress.2014.11.013", author = "R. Rocchetta and Y.F. Li and E. Zio" }
This work investigates new generalization error bounds on the predictive accuracy of Extreme Learning Machines (ELMs). Extreme Learning Machines are a special type of neural network that enjoy an extremely fast learning speed thanks to the convexity of the training program. This feature makes ELMs particularly useful to tackle online learning tasks. A new probabilistic bound on the accuracy of ELM is prescribed thanks to scenario decision-making theory. Scenario decision-making theory allows equipping the solutions of data-based decision-making problems with formal certificates of generalization. The resulting certificate bounds the probability of constraint violation for future scenarios (samples). The bounds hold non-asymptotically, distribution-free, and therefore quantify the uncertainty resulting from limited availability of training examples. We test the effectiveness of this new method on reliability-based decision-making problem. A data set of samples from the benchmark problem on robust control design is used for the online training of ELMs and empirical validation of the bound on their accuracy.
@InProceedings{ROCCHETTA_2021_ESREL, title = "New probabilistic guarantees on the accuracy of Extreme Learning Machines: an application to decision-making in a reliability context”", booktitle = "Proceedings of ESREL 2021 Conference", year = "2021", author = "R. Rocchetta" }
A method for constructing consonant predictive beliefs for multivariate datasets is presented. We make use of recent results in scenario theory to construct a family of enclosing sets that are associated with a predictive lower probability of new data falling in each given set. We show that the sequence of lower bounds indexed by enclosing set yields a consonant belief function. The presented method does not rely on the construction of a likelihood function, therefore possibility distributions can be obtained without the need for normalization. We present a practical example in two dimensions for the sake of visualization, to demonstrate the practical procedure of obtaining the sequence of nested sets
@InProceedings{ROCCHETTA_2021_ISIPTA, title = "Constructing consonant predictive beliefs from data with scenario theory", booktitle = "Proceedings of Machine Learning Research. ISIPTA Conference", year = "2021", author = "M. De Angelis, R. Rocchetta, A. Gray, S. Ferson" }
Scenario-based approaches to Reliability-Based Design-Optimization were recently proposed by the authors, Rocchetta et al. (2020). Scenario theory makes direct use of the available data thereby eliminating the need for creating a probabilistic model of the uncertainty in the parameters. This feature makes the resulting design exempt from the subjectivity caused by prescribing an uncertainty model from insufficient data. Most importantly, scenario theory renders a formally verifiable bound to the probability of failure. This bound is non-asymptotic and holds for any probabilistic model consistent with the available data. In this article we seek designs that minimize a combination of cost and penalty terms caused by violating reliability constraints. Similar to Conditional-Valueat- Risk programs, the proposed optimization approach is convex, thereby easing its numerical implementation. As opposite to a Conditional-Value-at-Risk method, a model for the uncertainty is not required and the method provides bounds on the reliability, which is valuable information to assess the robustness of the prescribed design. Furthermore, the proposed approach enables the analyst to shape the distribution of the design's performance according to a given value-at-risk. This is done by minimizing the empirical approximation of the integral of the design's performance in the loss/failure region. The effectiveness of the approach is tested on an easily reproducible numerical example with its strengths discussed in comparison to traditional methods.
@InProceedings{ROCCHETTA_2020_esrel, title = "An Empirical Approach to Reliability-based Design using Scenario Optimization", booktitle = "European Safety and Reliability Conference", year = "2020", author = "R. Rocchetta and L. G. Crespo" }
This article introduces a scenario optimization framework for reliability-based design given measurements of the uncertain parameters. In contrast to traditional methods, scenario optimization makes direct use of the available data thereby eliminating the need for assuming a distribution class and estimating its hyper-parameters. Scenario theory provides formal bounds on the probabilistic performance of a design decision and certifies the system ability to comply with various requirements for future/unseen observations. This probabilistic certificate of correctness is non-asymptotic and distribution-free. Furthermore, chance-constrained optimization techniques are used to detect and eliminate the effects of outliers in the resulting optimal design. The proposed framework is exemplified on a benchmark robust control challenge problem having conflicting design objectives.
@proceedings{10.1115/DSCC2019-8949, author = {Rocchetta, Roberto and Crespo, Luis G. and Kenny, Sean P.}, title = "{Solution of the Benchmark Control Problem by Scenario Optimization}", volume = {Volume 2: Modeling and Control of Engine and Aftertreatment Systems; Modeling and Control of IC Engines and Aftertreatment Systems; Modeling and Validation; Motion Planning and Tracking Control; Multi-Agent and Networked Systems; Renewable and Smart Energy Systems; Thermal Energy Systems; Uncertain Systems and Robustness; Unmanned Ground and Aerial Vehicles; Vehicle Dynamics and Stability; Vibrations: Modeling, Analysis, and Control}, series = {Dynamic Systems and Control Conference}, year = {2019}, month = {10}, abstract = "{This article introduces a scenario optimization framework for reliability-based design given measurements of the uncertain parameters. In contrast to traditional methods, scenario optimization makes direct use of the available data thereby eliminating the need for assuming a distribution class and estimating its hyper-parameters. Scenario theory provides formal bounds on the probabilistic performance of a design decision and certifies the system ability to comply with various requirements for future/unseen observations. This probabilistic certificate of correctness is non-asymptotic and distribution-free. Furthermore, chance-constrained optimization techniques are used to detect and eliminate the effects of outliers in the resulting optimal design. The proposed framework is exemplified on a benchmark robust control challenge problem having conflicting design objectives.}", doi = {10.1115/DSCC2019-8949}, url = {https://doi.org/10.1115/DSCC2019-8949}, note = {V002T24A001}, eprint = {https://asmedigitalcollection.asme.org/DSCC/proceedings-pdf/DSCC2019/59155/V002T24A001/6455518/v002t24a001-dscc2019-8949.pdf}, }
Cascading failures events are major concerns for future power grids and are generally not treat-able analytically. For realistic analysis of the cascading sequence, dedicated models for the numerical simulation are often required. These are generally computationally costly and involve many parameters and variables. Due to uncertainty associated with the cascading failures and limited or unavailable historical data on large size cascading events, several factors turn out to be poorly estimated or subjectively defined. In order to improve confidence in the model, sensitivity analysis is applied to reveal which among the uncertain factors have the highest influence on a realistic DC overload cascading model. The 95 th percentile of the demand not served, the estimated mean number of line failures and the frequency of line failure are the considered outputs. Those are obtained by evaluating random contingency and load scenarios for the network. The approach allows to reduce the dimensionality of the model input space and to identifying inputs interactions which are affecting the most statistical indicators of the demand not supplied.
@InProceedings{RocchettaESREL2018, author = {R. Rocchetta and E. Patelli and B. Li and G. Sansavini}, title = {Effect of Load-Generation Variability on Power Grid Cascading Failures}, booktitle = {European Safety and Reliability Conference (ESREL 2018)}, year = {2018}, }
The integration of renewable energy resources into our lives is vital for achieving a sustainable energy development. Renewable energy generation is undoubtedly an effective alternative for conventional electrical energy generation techniques, which are one of the key contributions for the emission of greenhouse gases. However, the introduction of renewable energy sources into the power grid is associated with significant costs. Here, an optimal localization and sizing of solar photovoltaic power generation plants in a power network are analyzed. Genetic algorithms are used to solve the optimization problem.
@INPROCEEDINGS{8398774, author={O. {Cangul} and R. {Rocchetta} and E. {Patelli} and M. {Fahrioglu}}, booktitle={2018 IEEE International Energy Conference (ENERGYCON)}, title={Financially optimal solar power sizing and positioning in a power grid}, year={2018}, volume={}, number={}, pages={1-6}, doi={10.1109/ENERGYCON.2018.8398774}}
In this work, we investigate Reinforcement Learning (RL) for managing Operation and Maintenance (O&M) of power grids equipped with Prognostic and Health Management (PHM) capabilities, which allow tracking the health state of the grid components. RL exploits this information to select optimal O&M actions on the grid components giving rise to state-action-reward trajectories maximising the expected profit. A scaled-down case study is solved for a power grid, and strengths and weaknesses of the framework are discussed.
@InProceedings{RocchettaREC2018, author = {R. Rocchetta and M. Compare and E. Patelli and E. Zio}, title = {A Reinforcement Learning Framework for Optimisation of Power Grid Operations and Maintenance}, booktitle = {workshop on Reliable Engineering Computing 2018}, year = {2018}, }
This paper presents a framework for stochastic analysis, simulation and optimisation of electric power grids combined with heat district networks. In this framework, distributed energy sources can be integrated within the grids and their performance is modelled. The effect of uncertain weather-operational conditions on the system cost and reliability is considered. A Monte Carlo Optimal Power Flow simulator is employed and statistical indicators of the system cost and reliability are obtained. Reliability and cost expectations are used to compare 4 different investments on heat pumps and electric power generators to be installed on a real-world grid. Generators' sizes and positions are analysed to reveal the sensitivity of the cost and reliability of the grid and an optimal investment problem is tackled by using a multi-objective genetic algorithm.
@InProceedings{8272792, author = {R. Rocchetta and E. Patelli}, title = {Stochastic analysis and reliability-cost optimization of distributed generators and air source heat pumps}, booktitle = {2017 2nd International Conference on System Reliability and Safety (ICSRS)}, year = {2017}, pages = {31-35}, month = {Dec}, doi = {10.1109/ICSRS.2017.8272792}, keywords = {Monte Carlo methods;distributed power generation;genetic algorithms;heat pumps;investment;power generation economics;power generation reliability;power grids;stochastic processes;Monte Carlo optimal power flow simulator;air source heat pumps;cost expectations;cost sensitivity analysis;distributed energy sources;distributed generators;electric power generators;electric power grids;generator sizes;heat district networks;multiobjective genetic algorithm;optimal investment problem;real-world grid;reliability-cost optimization;statistical indicators;stochastic analysis;system cost;uncertain weather-operational conditions;Cogeneration;Generators;Heat pumps;Power systems;Reliability;Resistance heating;heat pumps;interconnected grids reliability;renewable power;sensitivity;stochastic optimisation}, }
Add abstract here
@InProceedings{RocchettaESREL2017, author = {R. Rocchetta and E. Patelli}, title = {An Efficient Framework for Reliability Assessment of Power Networks Installing Renewable Generators and Subject to Parametric P-box Uncertainty}, booktitle = {Safety and Reliability. Theory and Applications (ESREL 2017)}, year = {2017}, publisher = {Taylor and Francs}, doi = {10.1201/9781315210469-411}, }